- Generative AI could unlock general-purpose robots by fusing skills.
- MIT’s “PoCo” approach uses diffusion models, boosting multi-task performance 20%.
- It combines real-world dexterity with simulation versatility.
Upgrading robot repertoire
For years, roboticists have fixated on hardware design while casually dropping the tantalizing term “general-purpose humanoids.”
However, the leap from single-task automatons to versatile all-rounders demands more than mechanical makeovers. Cue the entry of generative AI, which could revolutionize robotic intelligence and unleash the full potential of bipedal designs.
Devising effective training methods for multi-talented robots has been a persistent challenge. While reinforcement and imitation learning show promise, future solutions may blend these approaches, supercharged by generative AI models.
MIT researchers propose “policy composition” (PoCo), harnessing diffusion models to consolidate relevant info from task-specific datasets.
Skill fusion fiesta
PoCo trains individual diffusion models on distinct tasks, like pounding nails or flipping pancakes with a spatula. Then, it melds these “policies” into a unified strategy, enabling robots to juggle multiple tools and tackle unfamiliar jobs.
MIT reports a 20% performance boost using this method, including executing multi-step tasks seamlessly.
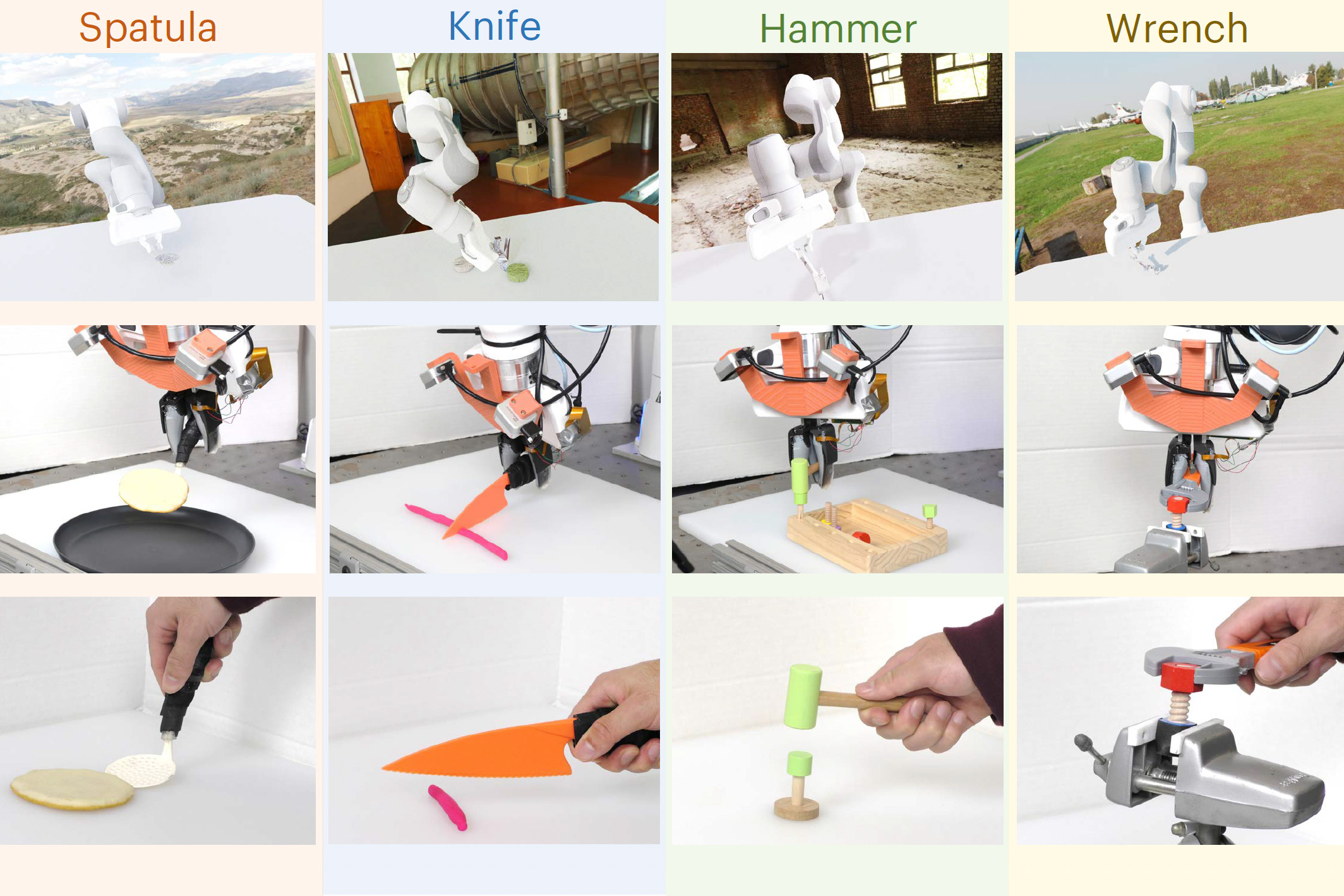
Intelligent systems that empower robots to swap tools on the fly, tackling diverse tasks with finesse. As Lirui Wang, the study’s lead author, puts it, “We can combine policies to get the best of both worlds” – real-world dexterity meets simulated adaptability.
This symbiosis edges us closer to that long-coveted “general-purpose” dream.