Tom is a Keynote Speaker at "All we have is now" and a cofounder of Interesting Speakers. Previously he was at Publicis Groupe where he headed Innovation.
Tom has over 700k followers on Linkedin and is a #1 Voice in Marketing on the platform.
Guest Author: Tom Goodwin
There is something people working at Open AI, Anthropic, Google etc, don’t want you to know, and that’s that they don’t really understand how their technology works.
They know THAT it works, they have theories, they understand the dynamics at play, but Large Language Models continually surprises them, for good at bad.
– LLM’s can render complex fluid dynamics as videos, but struggle to create images of hands.
– They can’t win at tic-tac-toe given options, but they can write the entire program to make tic-tac-toe and never lose.
– They can’t do basic maths often (even like multiplying two numbers) but they can infer and learn languages they’ve never been asked to learn, nor had data ingested to ever know.
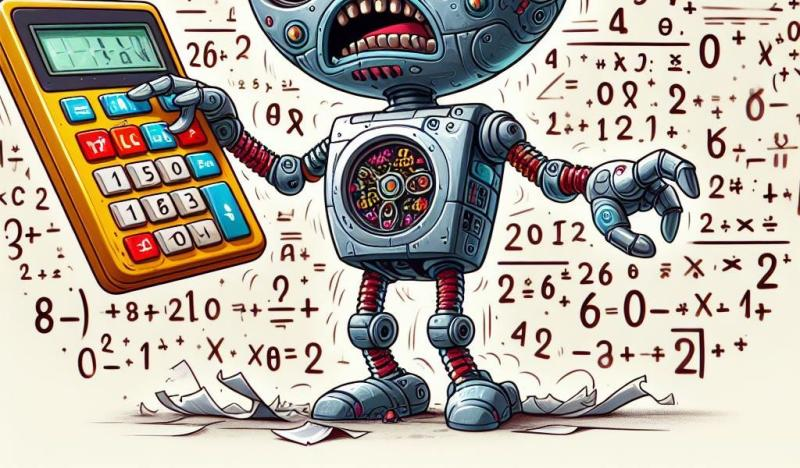
They do things that world experts find incredibly hard, and laborious and seem magical, while making mistakes a kid would be ashamed of.
And while some of the limitations are obvious, it’s a probability and relationship engine, it’s designed to always offer certainty, it’s unable to “understand’ ANYTHING, it’s subservient to a fault…….many of the things it excels and fails at, seem poorly explained and understood.
Which isn’t a problem per se, having a tech magical enough to be brilliant in ways we can’t predict, replicate or understand is rather exciting. Yet it does call into question three things we should consider.
1)How much does accuracy and reliability matter
In some fields being 70% accurate, 70% of the time, is absolutely fine, especially if there is a human in the loop. Writing a decent job spec, or PLU copy may not be that vital, in Drug discovery, only hits matter.
Some moments being 95% accurate, 95% of the time would be transformative, making advertising at scale, running training programs.
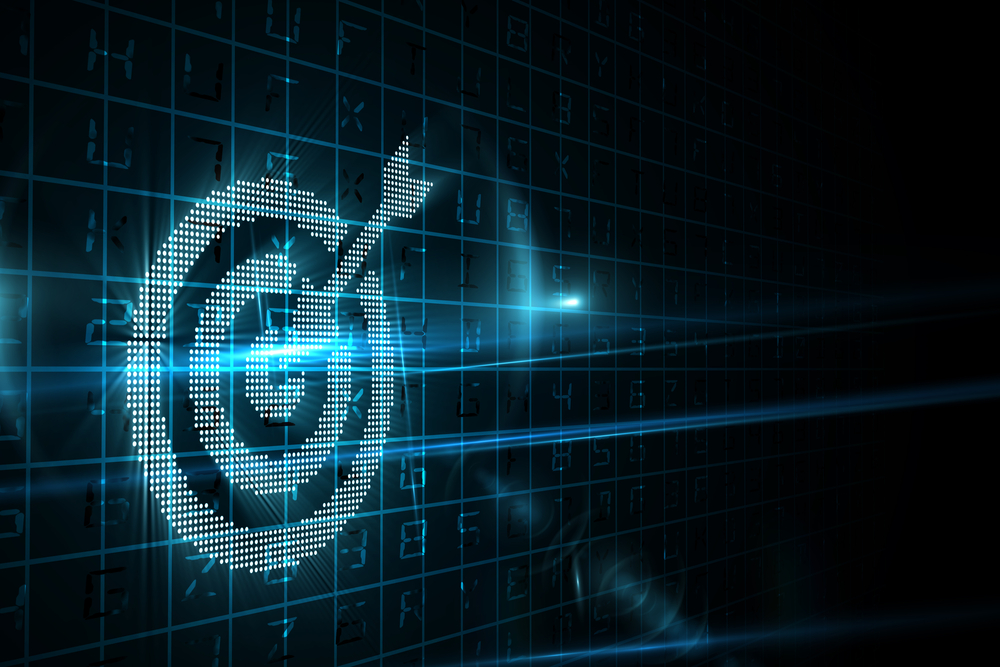
But quite often life needs things 99.9999% accurate,99.99999999% of the time or more.If you’re only messing up 1 person in 1,000’s cancer scans/tax returns/customer service/aircraft landing, it’s not especially helpful to point out how good it’s been the other time.
At what point does having humans in the loop make this OK, and when does it remove the efficiency.
2) Will it improve.
People are obsessed with the idea AI is the worst it’s ever going to be.
True, but which of these issues are easily cured by more data, more compute and more experience, and which are not issues of scale, but issues of construction.
If LLM’s are at the core based on probable combinations of data, pixels, words, numbers from the past, there are likely huge limitations to what scale solves. Unless it has a level of UNDERSTANDING one would imagine it won’t improve at many things enough, or quickly.
3) Do we get lazy?
For me the greatest threat from AI is that we slowly become lazy and useless. The better gets the more likely we had to care less. If you can reply to 99% of emails, brilliantly….why both checking them? And slowly but surely the construct of a company, the togetherness, the purpose, the sense of accomplishment dwindles.
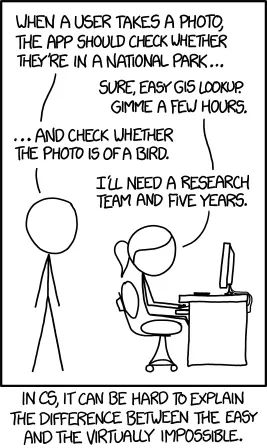